Benchopt
#
—Making your ML and optimization benchmarks simple and open—
Benchopt is a benchmarking suite tailored for machine learning workflows. It is built for simplicity, transparency, and reproducibility. It is implemented in Python but can run algorithms written in many programming languages.
Reproducing an existing benchmark should be as easy as running
benchopt run . --config ./example_config.yml
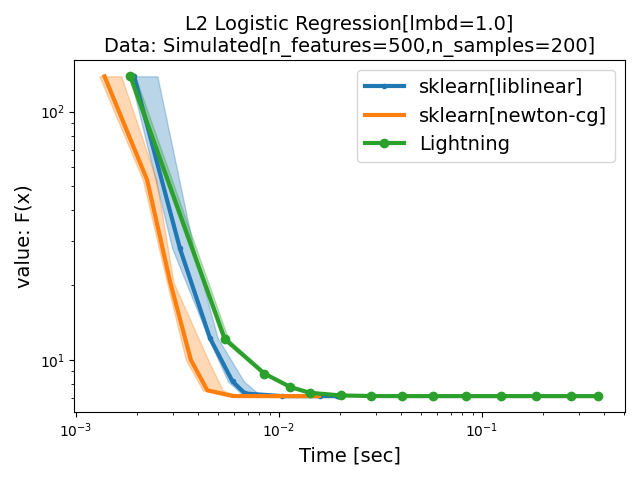
There are already many Available benchmarks that have been created using benchopt. Learn how to run them and how to construct your own with the following pages!
Get started
Install benchopt and run your first benchmark
Benchmark workflow
Write a benchmark from scratch, run it, visualize it, and publish it
Tutorials
Gallery of use-cases crafted by the benchopt community
User guide
Full documentation of benchopt API and CLI
Frequently asked questions (FAQ)#
How to add my solver to an existing benchmark?
Visit the Add a solver to an existing benchmark tutorial for a step-by-step procedure to add a solver to an existing benchmark.
How can I write a benchmark?
Learn how to Write a benchmark, including creating an objective, a solver, and a dataset.
Also take a look at our template repository for Optimization and ML to easily start a new benchmark.
How are performance curves constructed and the solvers stopped?
One of benchopt’s goals is to evaluate the method’s performance with respect to its computational budget. Benchopt allows several strategies to vary the computational budget, that can be set on a per solver basis. It is also possible to set various stopping criterions to decide when to stop growing the computational budget, to avoid wasting resources. Visit the Performance curves page for more details.
How can I reuse code in a benchmark?
For some solvers and datasets, it is handy to share some operations or pre-processing steps. Benchopt allows to factorize this code by Reusing some code in a benchmark.
Can I run a benchmark in parallel?
Benchopt allows to run different benchmarked methods in parallel, either with joblib
using -j 4
to run on multiple CPUs of a single machine or using SLURM, as described in Running the benchmark on a SLURM cluster.
Join the community#
Join benchopt discord server and get in touch with the community!
Feel free to drop a message to get help with running/constructing benchmarks or (why not) discuss new features to be added and future development directions that benchopt should take.
Citing Benchopt#
Benchopt is a continuous effort to make reproducible and transparent ML and optimization benchmarks. Join this endeavor! If you use benchopt in a scientific publication, please cite
@inproceedings{benchopt,
author = {Moreau, Thomas and Massias, Mathurin and Gramfort, Alexandre
and Ablin, Pierre and Bannier, Pierre-Antoine
and Charlier, Benjamin and Dagréou, Mathieu and Dupré la Tour, Tom
and Durif, Ghislain and F. Dantas, Cassio and Klopfenstein, Quentin
and Larsson, Johan and Lai, En and Lefort, Tanguy
and Malézieux, Benoit and Moufad, Badr and T. Nguyen, Binh and Rakotomamonjy,
Alain and Ramzi, Zaccharie and Salmon, Joseph and Vaiter, Samuel},
title = {Benchopt: Reproducible, efficient and collaborative optimization benchmarks},
year = {2022},
booktitle = {NeurIPS},
url = {https://arxiv.org/abs/2206.13424}
}